Labeling Tools for Machine Learning: A Comprehensive Guide
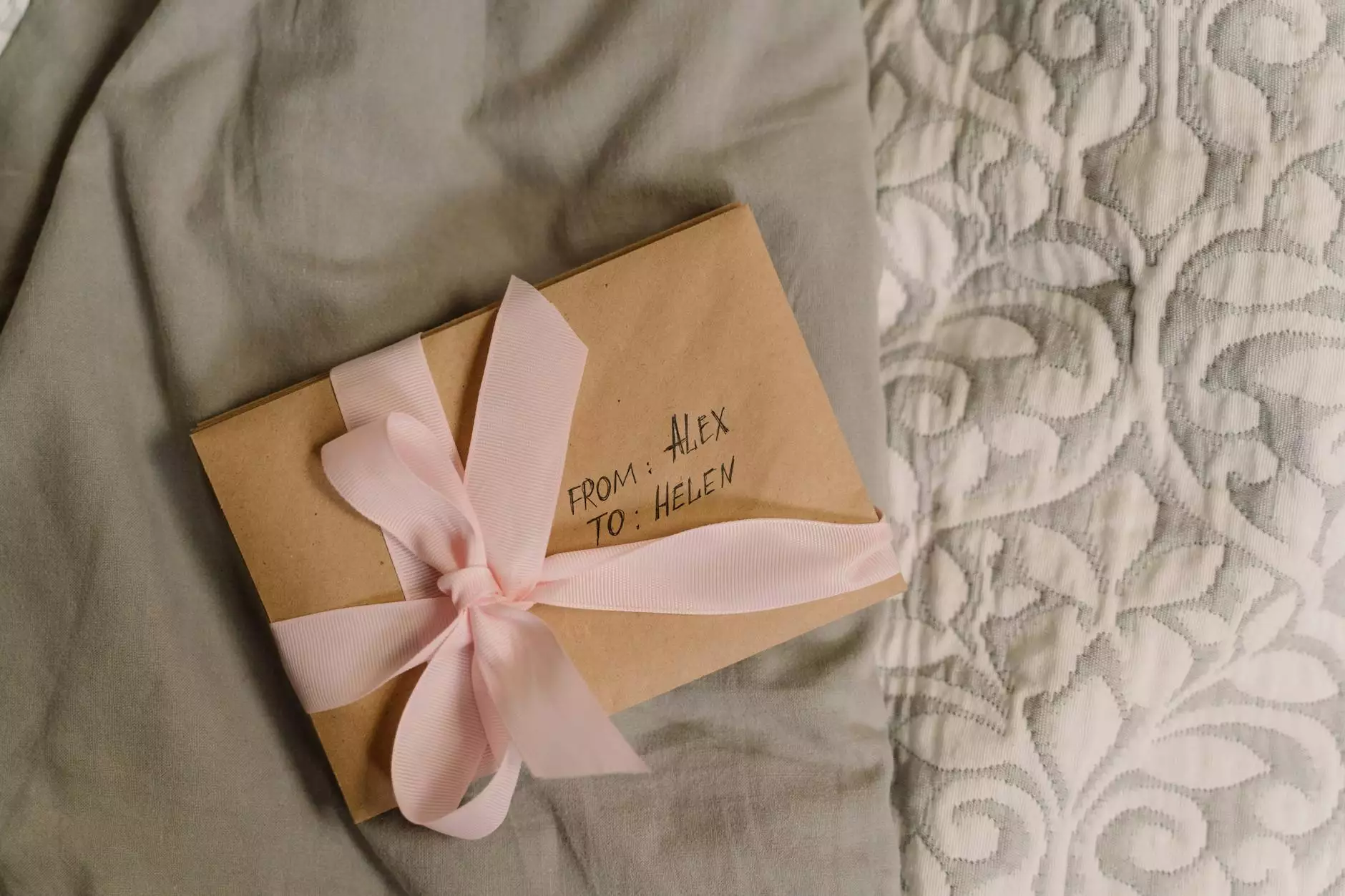
In the rapidly evolving world of technology, machine learning has emerged as a transformative force. From automation to intelligent decision making, the impact of machine learning is profound. However, a crucial step in deploying effective machine learning models is the proper labeling of data. This is where labeling tools for machine learning come into play. In this article, we will delve deeply into the fundamentals of data labeling, explore various tools available, and understand how to choose the right solution for your business needs.
What is Data Labeling?
Data labeling is the process of annotating data with informative tags, which enables the machine learning models to learn from it. This data can take various forms, such as text, images, audio, and video. Properly labeled data allows algorithms to make accurate predictions, enhancing model performance significantly. The quality of this labeling directly influences the effectiveness of the model, making this step fundamental in any machine learning process.
The Importance of Labeling in Machine Learning
Labeling in machine learning isn't just an optional step; it is essential for several reasons:
- Improved Accuracy: Properly labeled data leads to better and more accurate predictions.
- Model Training: Labeled datasets are critical for training algorithms by providing the correct answer to predictions.
- Data Validation: Well-labeled data helps in validating models during testing phases.
- Scalability: Using effective labeling tools allows for scalable operations in data processing and management.
Types of Labeling Tools for Machine Learning
Numerous labeling tools vary based on functionality, usability, and type of data being handled. Below are some common types of labeling tools:
1. Image Labeling Tools
These tools cater specifically to image datasets. They allow users to annotate images with bounding boxes, polygons, or segmentation masks. Commonly used image labeling tools include:
- Labelbox: A user-friendly interface with collaborative features.
- Supervisely: A robust platform for image annotation with advanced capabilities.
- VGG Image Annotator (VIA): An open-source tool suitable for simple annotation tasks.
2. Text Labeling Tools
Text labeling tools enable the annotation of text data. These tools are essential in natural language processing (NLP) applications, enabling sentiment analysis, entity recognition, and more. Popular text labeling tools include:
- Prodigy: An annotation tool powered by machine learning that enhances human input with algorithms.
- Label Studio: A versatile tool for text, image, and audio annotation.
- LightTag: A collaborative text annotation tool focusing on team efficiency.
3. Audio and Video Labeling Tools
These tools are essential for annotating audio and video data, crucial in applications like speech recognition and video analytics. Key tools for audio and video labeling include:
- Audiolabel: A simple tool for audio annotation with a straightforward interface.
- VideoLAN: A powerful multimedia tool that also allows video annotation.
- CVAT: A comprehensive video annotation tool that supports various formats and features.
Key Features to Look for in Labeling Tools
When selecting labeling tools for machine learning, certain features may significantly enhance your labeling process:
- User-Friendly Interface: An intuitive interface reduces the learning curve and enhances productivity.
- Collaboration Features: Tools that support multiple users allow teams to work together efficiently.
- Integration Capabilities: The ability to integrate with existing workflows and datasets is crucial for seamless operations.
- Automation Support: AI-driven suggestions can speed up the annotation process.
- Quality Control Mechanisms: Features such as consensus scoring and review processes ensure data accuracy.
Benefits of Using Labeling Tools
Utilizing dedicated labeling tools for machine learning provides several notable advantages:
1. Increased Efficiency
Automating parts of the labeling process with advanced tools can significantly reduce the time required for data preparation, enabling faster model development.
2. Enhanced Accuracy
Quality labeling tools often come with built-in validation features, ensuring that the annotations are consistent and correct.
3. Scalability
As data grows, efficient labeling tools allow businesses to scale their data preparation processes easily, managing larger datasets without compromising quality.
4. Cost-Effectiveness
Investing in labeling tools can lead to cost savings in the long run by reducing the need for extensive error-prone manual labeling, thereby increasing ROI.
Challenges in Data Labeling
Despite the numerous benefits, data labeling comes with its own set of challenges:
1. Manual Errors
Human annotators may introduce errors, particularly when labeling large datasets, thereby affecting model performance.
2. Time Constraints
Labeling can be a time-consuming process, especially for complex datasets, which may delay machine learning project timelines.
3. Subjectivity in Annotation
In some cases, labeling can be subjective, leading to inconsistencies in annotations that pose challenges for model accuracy.
Best Practices for Effective Data Labeling
To maximize the benefits of labeling tools for machine learning, adopting best practices is essential:
- Defining Clear Guidelines: Establish a clear labeling guideline to ensure uniformity in annotations across the dataset.
- Leveraging Automation: Use automation features offered by labeling tools to assist human annotators, which improves efficiency and accuracy.
- Regular Review Process: Implement a review system to validate labeled data and capture any inconsistencies early on.
- Training Labelers: Providing comprehensive training to labelers can improve the quality of annotations and reduce errors.
Conclusion
In conclusion, labeling tools for machine learning play a pivotal role in the success of machine learning projects. By enhancing the accuracy and efficiency of data labeling, businesses can significantly improve their model performance and outcomes. As you venture into the world of machine learning, selecting the right labeling tools tailored to your specific needs will be crucial for achieving your goals. Remember to consider the features mentioned above, along with best practices, to ensure that your data labeling process is as seamless and effective as possible.
For more detailed information and resources on machine learning and labeling tools, visit KeyMakr, where we specialize in providing top-notch services including Home Services and Keys & Locksmiths.