The Ultimate Guide to Data Labeling Platforms: Transforming Your Business
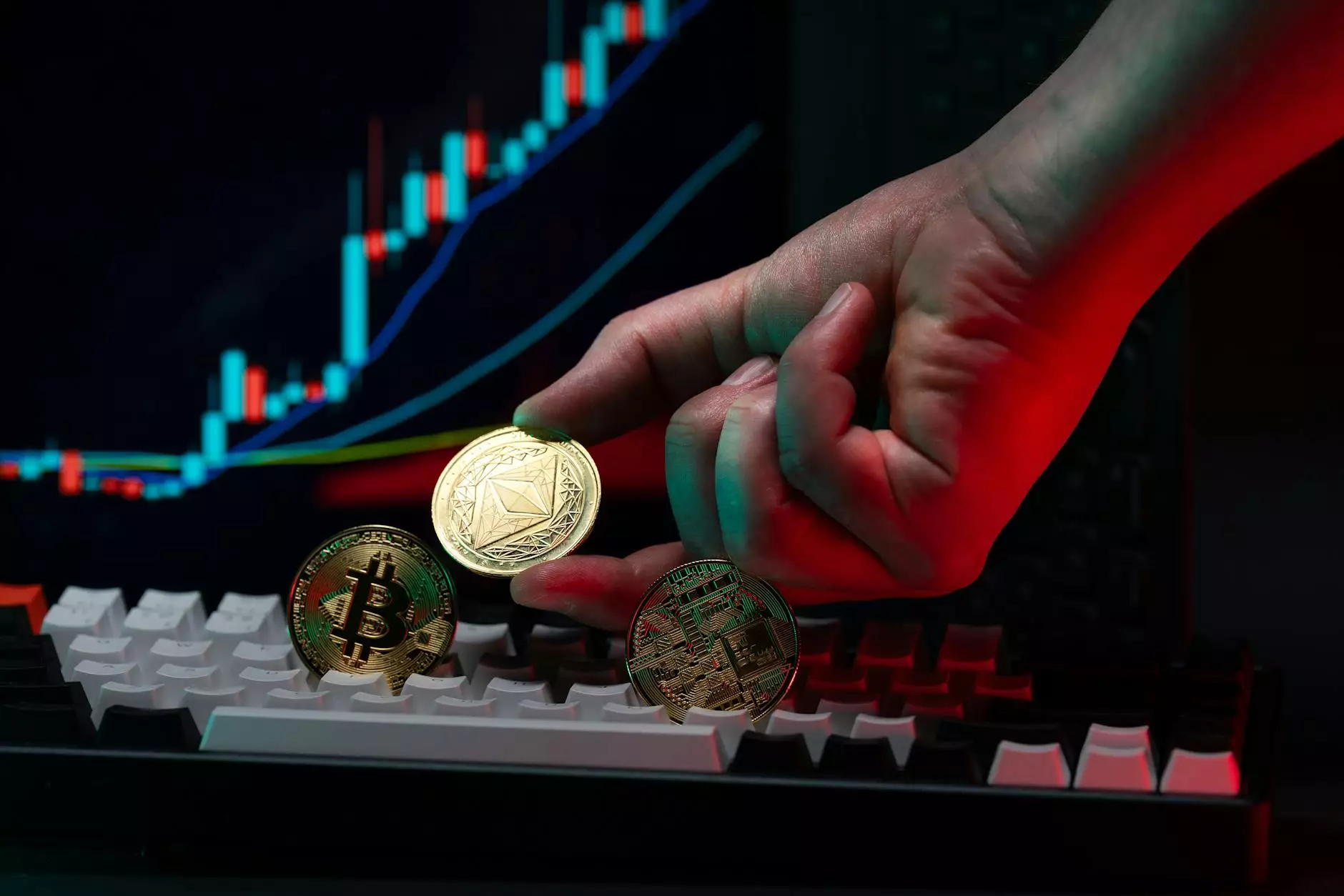
In the era of big data, businesses are constantly seeking innovative ways to leverage information for competitive advantage. One of the most critical components in this landscape is effective data management, particularly through the use of a data labeling platform. This article will delve into the significance of data labeling for software development, explore different types of labeling techniques, and discuss how platforms like Keymakr are at the forefront of revolutionizing data processes for businesses.
Understanding Data Labeling
Data labeling is the process of annotating data with tags that indicate the type of data or its characteristics. This is essential for training machine learning models, as computers need clear guidance to understand the context of information. Here’s how data labeling directly impacts your business:
- Improved Model Accuracy: Well-labeled data leads to more reliable predictions.
- Faster Deployment: Streamlined data preparation means quicker implementation of machine learning projects.
- Enhanced Insights: Annotated data allows for better analysis and reporting, helping businesses make informed decisions.
Why Use a Data Labeling Platform?
Utilizing a data labeling platform offers several advantages over traditional methods of data organization and labeling:
1. Efficiency and Scalability
In high-volume data environments, manual labeling can be time-consuming and prone to error. A dedicated data labeling platform automates many of these processes, increasing efficiency and scalability. Keymakr’s platform, for instance, integrates AI tools to assist in the labeling process, allowing teams to handle larger datasets without compromising quality.
2. Cost-Effectiveness
Investing in a data labeling platform can significantly reduce costs over time. Automating labeling tasks lessens the need for extensive human resources and minimizes operational delays. By using Keymakr, businesses can ensure they are maximizing their return on investment (ROI) through optimized resource allocation.
3. Collaboration and Integration
A premier data labeling platform supports collaboration across teams and departments. Keymakr’s software not only facilitates internal collaboration but also seamlessly integrates with popular data management tools, ensuring that your workflow is uninterrupted and synchronized.
The Process of Data Labeling
The data labeling process typically includes the following steps:
- Data Collection: Gather raw data from various sources relevant to your business needs.
- Data Preprocessing: Clean and prepare the data to ensure accuracy and consistency.
- Labeling: Annotate the data using a combination of automated and manual methods to ensure thoroughness.
- Quality Assurance: Validate the labeled data to eliminate errors.
- Deployment: Implement the labeled data into your machine learning models or analytics tools.
Types of Data Labeling Techniques
Different projects require different labeling techniques. Here are some prevalent methods used in the industry:
1. Image Annotation
This technique is critical in fields such as computer vision. Image annotation involves marking objects within images with bounding boxes, polygons, or segmentation masks. This type of labeling is essential for training neural networks in applications like self-driving cars and facial recognition.
2. Text Annotation
Text data is abundant, and effective labeling can enhance natural language processing (NLP) tasks. Text annotation includes techniques such as sentiment analysis, where texts are categorized based on emotional tone, or entity recognition, where specific terms or phrases are tagged with relevant labels.
3. Video Annotation
With video content on the rise, labeling video data has become an essential part of training models in surveillance, sports analytics, and more. Techniques include frame-by-frame labeling and tracking moving objects through sequences.
Choosing the Right Data Labeling Platform
When selecting a data labeling platform, several factors should be considered to ensure it aligns with your business objectives:
1. User-Friendly Interface
A platform with an intuitive design enables users to navigate the software seamlessly, which is crucial for enhancing productivity among team members.
2. Flexibility and Customization
Different projects often require unique features. A good data labeling platform should allow for customization to adapt to various labeling requirements specific to your business or industry.
3. AI and Automation Features
As mentioned earlier, the integration of AI can dramatically boost the efficiency of the labeling process. Look for platforms that offer machine learning capabilities to streamline repetitive tasks.
4. Quality Control Mechanisms
Robust quality assurance features are vital to ensure the accuracy and reliability of labeled data. Choose a platform that provides tools for reviewing and validating labeled data efficiently.
Case Studies: Success Stories with Data Labeling Platforms
1. E-commerce Platform
An emerging e-commerce brand utilized Keymakr's data labeling platform to enhance its product categorization. By automating the labeling of product images and descriptions, they improved their search functionality, leading to a 30% increase in conversion rates over three months.
2. Autonomous Vehicle Company
A leading autonomous vehicle developer turned to Keymakr for efficient image annotation of their training datasets. With the platform’s AI-facilitated annotation capabilities, the company reduced their labeling time by 50%, enabling faster iteration cycles and improved model performance.
Future Trends in Data Labeling
As technology continues to evolve, so too will the methodologies and tools associated with data labeling. Some anticipated trends include:
- Increased Use of Synthetic Data: Generating synthetic datasets will become more common, helping to mitigate privacy concerns while expanding the variety of training data.
- Integration of Augmented Reality (AR): The incorporation of AR in data annotation processes may enhance accuracy and reliability, particularly in complex environments.
- Advanced Collaboration Tools: New collaborative tools will further enhance team interactions within data labeling environments, promoting efficiency and quality.
Conclusion: The Strategic Role of Data Labeling Platforms in Business
In an increasingly data-driven world, leveraging a data labeling platform is not just beneficial; it is essential. Implementing such a solution can drastically improve the quality of your data processing, facilitate better decision-making, and foster innovation within your business. Organizations looking to harness the full power of their data should consider platforms like Keymakr to optimize their data labeling processes and ensure a competitive edge in the marketplace.